The first chapter of Judea Pearl’s book, titled “Mind over Data,” strikes a chord with me so profoundly that I am almost tempted to etch it into my skin, though I acknowledge that might be an exaggeration. The chapter underscores the critical importance of reasoning in an era where we often place undue trust in data. Simply amassing large volumes of data does not absolve us of the need for thoughtful analysis, especially in contexts that are inherently non-deterministic. This responsibility goes beyond a mere task; it is a fundamental duty we must fulfill.
The concept of “Big Data” has risen to prominence, becoming a tantalizing addition to resumes. However, it demands a cautious approach. The advent of smartphones, which collect vast amounts of data daily, has necessitated not only the collection but also the effective use of this information. As a result, powerful computers and sophisticated models like machine learning have become central to addressing these needs. This shift has spurred a growing demand for computer science professionals known as data scientists, who specialize in managing and exploiting these data troves. The publishing of numerous models in academic journals and the popularization of technologies like GPT Chat illustrate this trend. Yet, as we delve deeper into these developments, concerns about their potential dangers surface, including instances where machine learning-based devices have caused both physical and ethical harm, prompting a reevaluation of their safety.
Enhancing the safety of these algorithms involves addressing several key areas: data quality, explainability, and the generalizability of the models. Data quality and availability are critical for the performance and reliability of AI systems. High-quality, diverse, and representative datasets are essential for effective learning and making accurate predictions. Yet, collecting data can be fraught with challenges; datasets may be incomplete, contain errors, or carry biases that do not accurately reflect real-world scenarios. Models built on flawed assumptions can lead to biased or unfair outcomes. Therefore, ensuring data quality and mitigating biases are imperative to avoid discriminatory results and ethical dilemmas.
Explainability is another vital component. AI models often encapsulate complex relationships within data that are not readily interpretable by humans. The complexity of deep learning models, characterized by millions of parameters and layers, can obscure the understanding of how decisions are made. This lack of transparency compromises accountability, error identification, and safety assurance. It becomes challenging to diagnose problems, justify decisions, and build trust in the systems.
Generalizability refers to the ability of AI systems to perform effectively on new, unseen data beyond the training set. A lack of generalizability can lead to incorrect decisions, difficulty in adapting to new situations, amplification of biases, security vulnerabilities, and reduced accountability. It is crucial that AI models can generalize across different contexts and handle unexpected scenarios to ensure their safe and reliable deployment.
Addressing these challenges necessitates the engagement of the human mind. Counterfactual thinking, a unique capability of human cognition, is crucial. By employing counterfactual reasoning, humans can anticipate risks, identify biases, test robustness, analyze failures, and consider ethical dimensions. Counterfactual questions encourage us to think about hypothetical scenarios, challenge underlying assumptions, and identify possible improvements. This type of thinking enhances critical analysis, deepens our understanding of causality and context, and fosters creative problem-solving. Without tapping into the human mind’s ability for counterfactual thinking, we cannot effectively address the shortcomings in AI systems, including issues with generalizability and data quality.
In conclusion, the theme “mind over data” serves as a potent reminder of the indispensable role of human cognition in the domain of AI. While data is invaluable, our dependency on it should not overshadow the necessity for reasoning, analysis, and critical thinking. Merely acquiring large amounts of data does not relieve us of the duty to thoroughly understand and interpret it. We must recognize that the human mind, with its capacity to process and make sense of complex information, remains an essential asset in ensuring the safety and ethical application of AI systems.
In summary, securing the safety of ML algorithms demands attention to data quality, explainability, and generalizability. The inherent counterfactual thinking within the human mind is vital for recognizing risks, exposing biases, assessing robustness, analyzing failures, and contemplating ethical concerns. By embracing this cognitive ability, we can develop and deploy AI systems that are not only safer and more reliable but also aligned with human values.
.
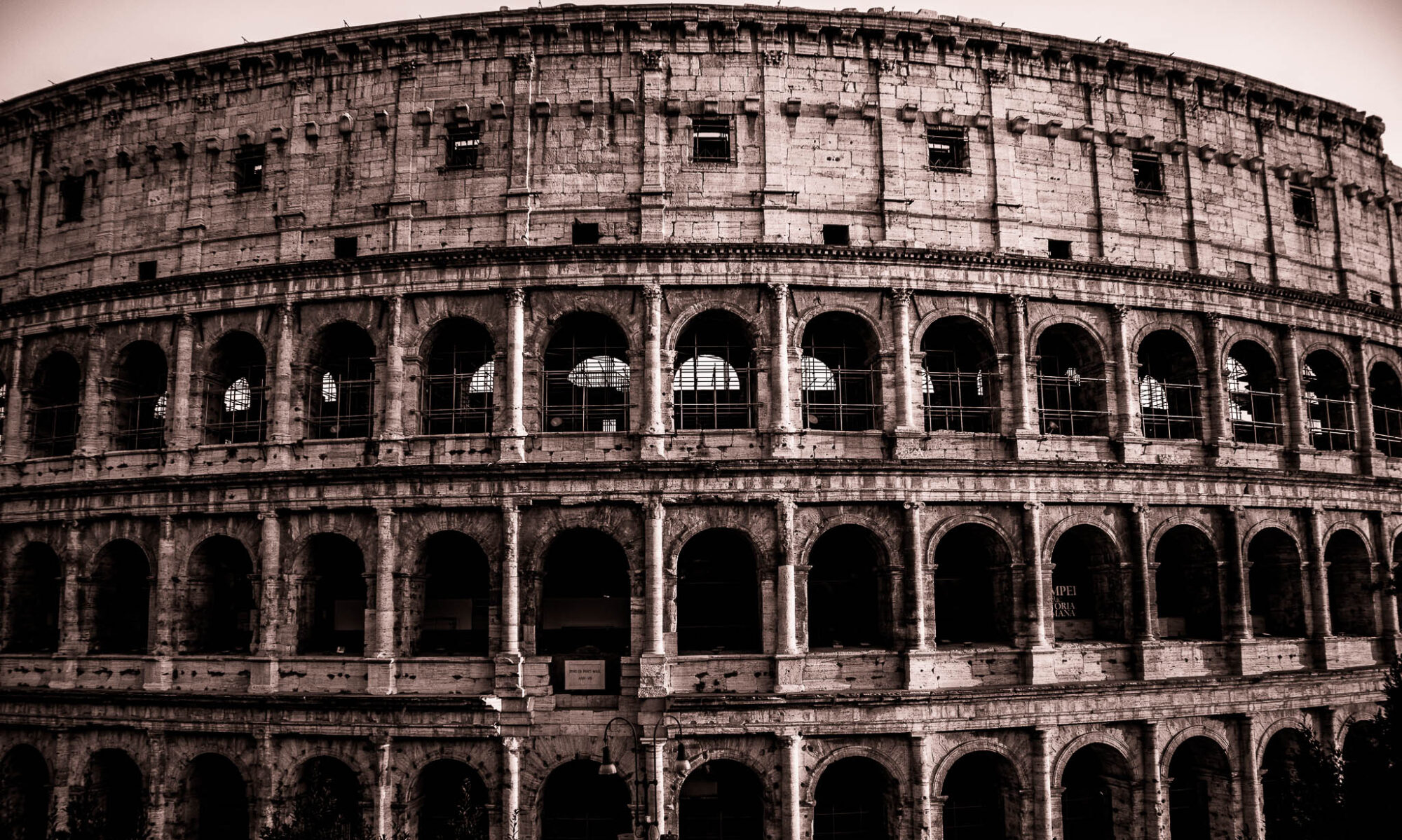